Searching for a job on a job board can be a frustrating experience. Current job search platforms rely heavily on keyword-based searches, which often produce irrelevant results, leaving both job seekers and employers dissatisfied. However, the landscape is changing, thanks to AI-powered technologies like vector databases, embeddings, and machine learning models that are poised to revolutionize how we connect people with the right opportunities. In this blog, we’ll explore the limitations of traditional job search systems, the emerging AI-driven solutions, the benefits they offer, the potential costs, and what the future holds.
The Shortcomings of Current Job Search Systems
Today’s job search platforms are dominated by keyword-based systems. These platforms typically match job seekers to openings based on the presence of certain terms in resumes and job descriptions. While this seems straightforward, it’s actually rife with issues. Here are some of the key limitations:
- Inaccurate Matches: Job seekers often encounter job postings that don’t quite fit their skills or experience, even though the keywords align. For example, someone searching for a “Python Developer” role may be greeted with listings for a Zookeeper Specializing in Reptiles or even a Biologist Studying Pythons in the Amazon. While both jobs involve “Python,” they probably weren’t what the candidate had in mind!
- Lack of Context: Traditional search engines struggle to understand the context behind a search query. If you’re looking for a “Marketing Ninja,” you might end up seeing jobs like Karate Instructor or Costume Designer for Ninja-Themed Movies—positions that, while fun, probably don’t align with your digital marketing aspirations.
- Overlooked Talent: Candidates with non-traditional career paths or resumes that don’t use “industry-standard” keywords can be overlooked, even if they’re highly qualified for a role.
- Difficulty with Synonyms and Variations: A search for “software engineer” may not yield relevant results if the job posting uses “developer” instead. The rigid structure of current systems struggles to handle variations in job titles, skill descriptions, or even location preferences.
The Shift to AI-Powered Search and Match Solutions
AI-powered search and match solutions are emerging as a game-changer in the world of job search by addressing the key challenges of traditional systems. With the integration of vector databases, embeddings, and machine learning, these technologies are solving the inefficiencies of keyword-based search methods.
In the world of modern information retrieval, traditional keyword-based searches often fall short when it comes to capturing the nuances of language and context. This is where semantic search comes into play. Unlike traditional methods that rely on exact keyword matches, semantic search aims to understand the meaning behind words and phrases, providing contextually relevant results. AI-powered systems leverage embeddings and vector databases to achieve this.
Embeddings are the backbone of Semantic understanding. There are numerical representations of words or phrases in a continuous vector space. They are a core technology that enables AI-powered job search systems to understand the context of job titles, skills, and job descriptions. These representations are created through techniques which train on massive text datasets to capture the relationships between words.
For example, in a word embedding space, terms like “software engineer” and “developer” would be placed close to each other because of their semantic similarity. This allows the system to match a user’s search for “software engineer” with job postings that might use “developer” instead, delivering more accurate results.
Vector databases—also known as similarity search databases—are designed to store and efficiently query vector representations like embeddings, and help optimize the search process. Instead of relying on traditional keyword matching, these databases compare the similarity between the vector of a user’s query (e.g., “data scientist in New York”) and the vectors representing job postings stored in the database. This approach significantly reduces computational load and speeds up the search process.
By integrating vector databases into job search platforms, the system can rapidly identify similar job postings, even when the exact keywords differ. This is a huge leap forward in delivering relevant job matches based on the actual meaning of the query, rather than just its literal content.
Benefits of AI-Powered Job Search
The shift to AI-powered job search systems brings several key benefits that make the job search process more efficient and user-friendly:
- Improved Matching Accuracy: By using semantic search powered by embeddings and vector databases, AI-powered platforms can deliver far more relevant job matches. Users receive results that better align with their skills, experience, and preferences, even if the exact wording differs between their resume and the job description.
- Faster Job Search: Vector databases allow for rapid comparisons between a user’s query and job listings, dramatically speeding up the search process. Job seekers no longer need to wade through irrelevant listings to find the right match.
- More Inclusive Searches: Traditional keyword-based searches often miss out on qualified candidates who don’t use the “right” terminology. AI systems, by understanding the meaning behind words, can help surface diverse talent, even those with non-traditional career paths.
- Personalized Experiences: As job seekers interact with the platform, machine learning models learn their preferences and behavior, allowing for continuous improvement in search results. Over time, these systems can deliver increasingly personalized job recommendations, with or without, and AI Agent.
Costs and Considerations
While AI-powered job search systems offer significant benefits, there are also some costs and challenges involved in implementing these technologies:
- Development and Integration Costs: Building an AI-powered search system involves substantial upfront investment in data science expertise, machine learning infrastructure, and vector databases. The cost of development can be significant, especially for smaller companies.
- Data Quality and Privacy: AI systems rely on large amounts of high-quality data to function effectively. Poor data quality can lead to inaccurate results, and handling sensitive user data—such as resumes and personal details—requires strict privacy and security measures to ensure compliance with regulations like GDPR.
- Ongoing Maintenance and Model Tuning: AI-powered platforms require continuous monitoring, tuning, and retraining to maintain performance. As job titles, skills, and market trends evolve, the system must adapt, adding to operational costs.
- Transparency and Trust: Users may be hesitant to trust AI systems, especially if the decision-making process is not transparent. It’s important to ensure that the system provides clear and explainable results to build user confidence in the technology.
Looking Ahead: The Future of AI-Powered Job Search
As AI technologies like embeddings and vector databases continue to evolve, the future of job search looks increasingly intelligent and personalized. AI-powered platforms are enabling more accurate and efficient job matching, reducing the frustration of irrelevant search results and missed opportunities. In the coming years, we can expect these systems to become even more powerful, integrating deeper levels of personalization and offering real-time feedback loops to continually refine job recommendations.
The key to success will be balancing technological innovation with user experience, ensuring that AI tools are not only effective but also easy to use and understand. Ultimately, AI-powered job search is not just about faster or better matches—it’s about fundamentally reimagining how we connect people with meaningful work.
By embracing emerging AI technologies like vector databases and embeddings, job search platforms are transforming into smarter, more intuitive systems that finally address the shortcomings of traditional methods. It’s an exciting time for job seekers and employers alike, as AI reshapes the future of job search and talent matching.
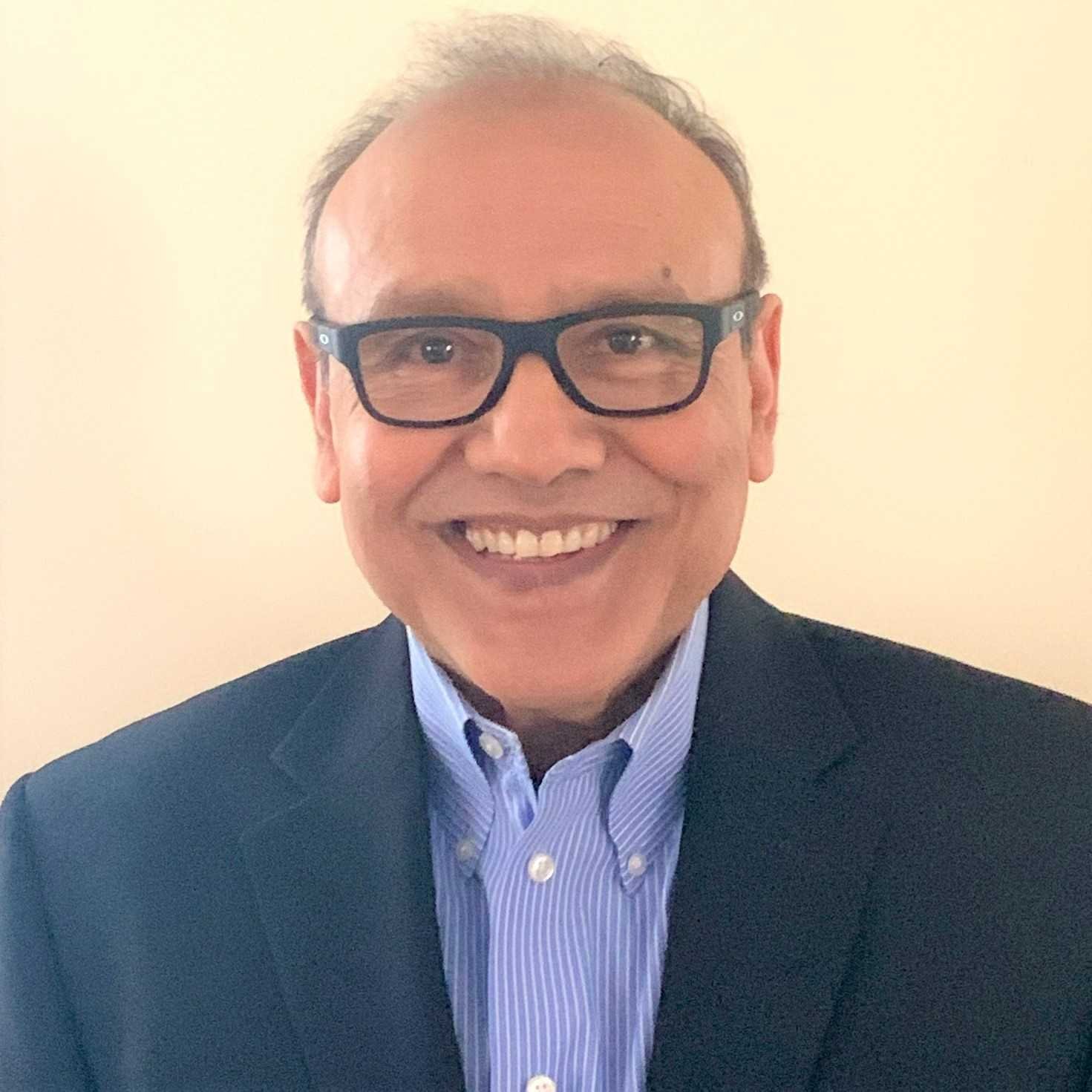
More Blogs From Rathin Sinha
Read Company Blog